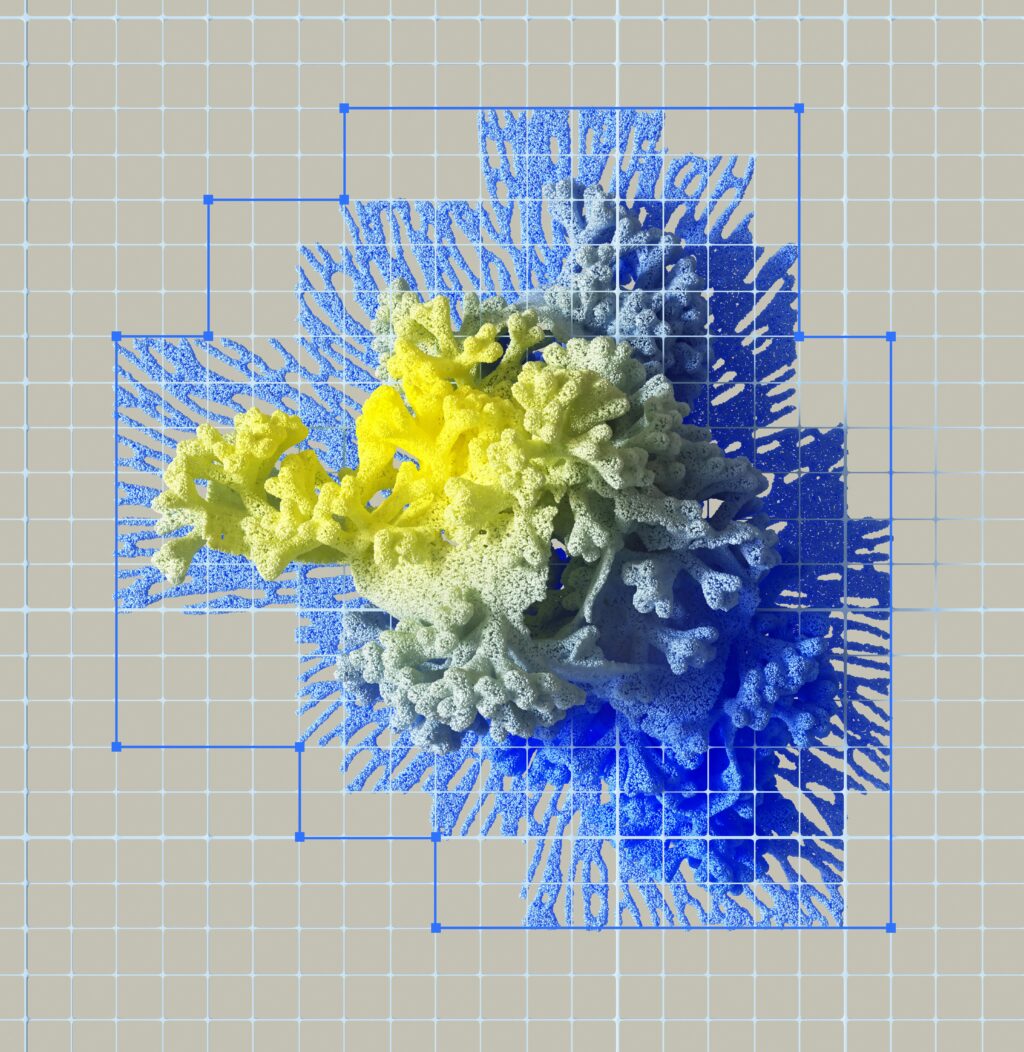
In today’s tech world, machine learning (ML) is more than just a catchphrase, it can be the answer to unlocking the secrets that lie within our DNA. What if the risk of cancer, diabetes, or heart disease could be foreseen by a straightforward DNA analysis? This is the promise of Polygenic Risk Scores (PRS), yet it’s not quite science fiction. These scores estimate a person’s susceptibility to disease by analysing the cumulative influence of many genetic variations. Despite its potential, PRS’s precision and reliability have been constrained by the complexity of genetic data. Machine learning, or ML for short, is a game-changer that improves PRS’s accuracy and adaptability to a variety of demographics.
In this blog, we’ll explore how ML is transforming the calculation of PRS, making it a powerful tool for personalized medicine.
Understanding Polygenic Risk Scores
Polygenic Risk Scores compile information from thousands or even millions of genetic variants, each of which contributes to a small effect on a person’s likelihood of developing a particular disease. For example, PRS identifies genetic markers, such as those associated with lipid metabolism or inflammatory responses, to calculate the likelihood of developing conditions like cardiovascular disease or type 2 diabetes. By analyzing a person’s DNA for a collection of variants related to high cholesterol, a PRS can predict their risk of developing cardiovascular disease.
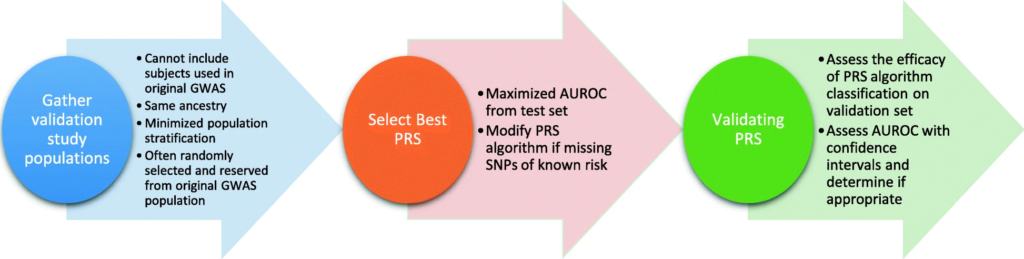
However, calculating PRS isn’t that straightforward. Traditional methods often oversimplify the complexity of genetic interactions and generally fail to integrate non-genetic factors like lifestyle and environment. That’s where ML steps in, refining the analysis to uncover hidden insights.
How Machine Learning Enhances PRS
Machine learning significantly enhances polygenic risk scores (PRS) by improving the way we analyze genetic data. Usually, traditional PRS relies on genome-wide association studies (GWAS) to identify genetic variants associated with diseases, but machine learning takes this further by uncovering subtle interactions between variants that GWAS might miss. For example, algorithms like random forests and support vector machines can identify combinations of variants that work together to influence disease risk, offering a deeper understanding of genetic factors.
Another way machine learning improves PRS is by handling the massive size of genomic datasets. With millions of variants to consider, these datasets can be overwhelming. ML algorithms use techniques like dimensionality reduction to condense the data, keeping only the most important information. Methods like Principal Component Analysis (PCA) and autoencoders make the analysis more efficient and accurate, ensuring we don’t lose valuable insights in such large amounts of data. Machine learning also allows us to take a broader approach by integrating multiple types of data beyond just genetic information. Diseases are rarely caused by genetics alone, epigenetic factors, gene expression levels, and even metabolic profiles all play a part. By combining these different data types into one model, machine learning gives us a more complete picture of disease risk. For example, when transcriptomic data is added to genomic data, it can improve predictions, like those for cancer risk.
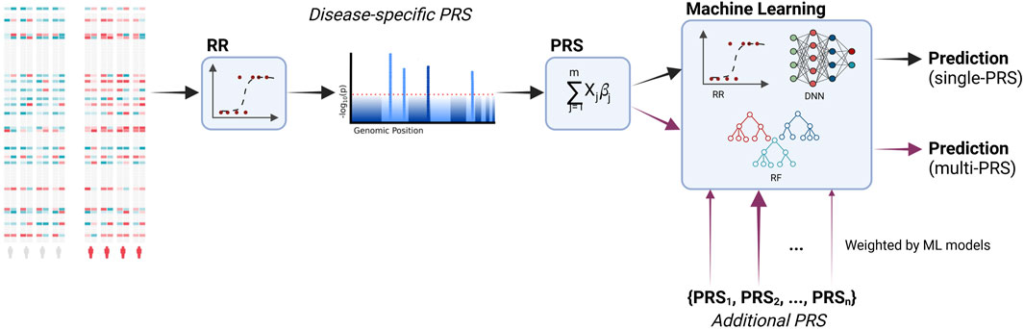
Finally, machine learning enables more personalized predictions. Traditional PRS tends to rely on population-level averages, but machine learning can take into account individual factors like age, lifestyle, and environment. Algorithms such as XGBoost and neural networks refine risk scores to better suit each person, leading to more accurate and actionable health interventions. This personalized approach is key to making healthcare not just more precise, but also more relevant to each individual.
Machine learning (ML)-enhanced polygenic risk score (PRS) models have been applied in various fields to improve risk predictions and enable more targeted interventions. In the case of heart disease, which remains a leading cause of death worldwide, ML-enhanced PRS models have improved the accuracy of risk predictions by combining genetic data with biomarkers like cholesterol levels and lifestyle factors such as diet and exercise. A case study from the UK Biobank demonstrated significant improvements in early identification of at-risk individuals, enabling timely interventions. In oncology, PRS refined by ML has shown promise in predicting risks for various cancer types. Deep learning models trained on genomic and transcriptomic data have achieved remarkable accuracy, even identifying rare genetic interactions that traditional methods miss. These models have also facilitated early detection and better stratification of patients for clinical trials. ML-enhanced PRS has been pivotal in improving predictions for Type 2 diabetes. By integrating genetic variants with lifestyle data such as diet, exercise, and BMI, models achieved a 15% higher accuracy than traditional approaches. This has allowed for better-targeted lifestyle interventions to reduce disease onset.
Challenges include the fact that most genomic studies have predominantly used data from individuals of European ancestry. ML models trained on such datasets may perform poorly for other populations. Increasing diversity in genomic research is essential to ensure equitable healthcare. Many ML models act as “black boxes,” making it difficult to understand how they arrive at predictions. Efforts like Explainable AI (XAI) are addressing this issue by providing insights into model decision-making. The use of genetic data raises questions about privacy, consent, and potential misuse, such as genetic discrimination in insurance or employment. Clear regulations and robust data protection measures are critical. Training ML models on genomic data requires significant computational resources, often necessitating high-performance computing (HPC) or cloud-based solutions. Access to these resources can be a limiting factor for smaller research institutions.
The future of ML in polygenic risk scores (PRS) is looking very promising, with several key advancements on the horizon. One of these is federated learning, which helps address privacy concerns by allowing ML models to be trained across multiple datasets without sharing sensitive data between institutions. This decentralized approach keeps data secure while encouraging collaboration across research and healthcare organizations. Another important development is improving the interpretability of ML models, which is crucial for making them more practical in clinical settings. Methods like SHAP (SHapley Additive exPlanations) are making it easier for researchers and doctors to understand and trust the predictions made by these models. This makes ML-powered PRS much more useful in real-world healthcare, where clear explanations are needed to make decisions based on risk scores. Looking ahead, we’re also getting closer to the idea of real-time risk assessments. Imagine going to your doctor and, right then and there, receiving a risk assessment based on your DNA and lifestyle. Thanks to advances in ML, this could soon become a reality, allowing people to make better-informed health decisions in the moment. This could truly change how we approach healthcare and prevention, making it much more personalized and immediate.
In conclusion, machine learning is revolutionizing how we calculate and apply Polygenic Risk Scores. By making them more accurate, personalized, and inclusive, machine learning (ML) is paving the way for a new era in precision medicine. The integration of ML and PRS has the potential to transform healthcare, offering tailored interventions that can save lives and improve quality of life. As we overcome challenges like data diversity and ethical concerns, the future of ML-enhanced PRS looks quite promising.
References
- O’Sullivan JW, Raghavan S, Marquez-Luna C, Luzum JA, Damrauer SM, Ashley EA, O’Donnell CJ, Willer CJ, Natarajan P; American Heart Association Council on Genomic and Precision Medicine; Council on Clinical Cardiology; Council on Arteriosclerosis, Thrombosis and Vascular Biology; Council on Cardiovascular Radiology and Intervention; Council on Lifestyle and Cardiometabolic Health; and Council on Peripheral Vascular Disease. Polygenic Risk Scores for Cardiovascular Disease: A Scientific Statement From the American Heart Association. Circulation. 2022 Aug 23;146(8):e93-e118. doi: 10.1161/CIR.0000000000001077. Epub 2022 Jul 18. PMID: 35862132; PMCID: PMC9847481.
- Slunecka, J.L., van der Zee, M.D., Beck, J.J. et al. Implementation and implications for polygenic risk scores in healthcare. Hum Genomics 15, 46 (2021). https://doi.org/10.1186/s40246-021-00339-y
- Lugner, M., Rawshani, A., Helleryd, E. et al. Identifying top ten predictors of type 2 diabetes through machine learning analysis of UK Biobank data. Sci Rep 14, 2102 (2024). https://doi.org/10.1038/s41598-024-52023-5
- Ahlberg, G., Andreasen, L., Ghouse, J., Bertelsen, L., Bundgaard, H., Haunsø, S., Svendsen, J. H., & Olesen, M. S. (2021). Genome-wide association study identifies 18 novel loci associated with left atrial volume and function. European Heart Journal, 42(44). https://doi.org/10.1093/eurheartj/ehab466
- Choi, S. W., Shin, T., Mak, H., & O’reilly, P. F. (2018). A guide to performing Polygenic Risk Score analyses. BioRxiv.
- Duan, Q., Hu, S., Deng, R., & Lu, Z. (2022). Combined Federated and Split Learning in Edge Computing for Ubiquitous Intelligence in Internet of Things: State-of-the-Art and Future Directions. Sensors, 22(16). https://doi.org/10.3390/s22165983
- Euesden, J., Lewis, C. M., & O’Reilly, P. F. (2015). PRSice: Polygenic Risk Score software. Bioinformatics, 31(9). https://doi.org/10.1093/bioinformatics/btu848
- He, X., Li, S., He, X. T., Wang, W., Zhang, X., & Wang, B. (2022). A Novel Ensemble Learning Model Combined XGBoost With Deep Neural Network for Credit Scoring. Journal of Information Technology Research, 15(1). https://doi.org/10.4018/jitr.299924
- Klau, J. H., Maj, C., Klinkhammer, H., Krawitz, P. M., Mayr, A., Hillmer, A. M., Schumacher, J., & Heider, D. (2023). AI-based multi-PRS models outperform classical single-PRS models. Frontiers in Genetics, 14. https://doi.org/10.3389/fgene.2023.1217860
- Liu, X., Tong, X., Zhu, J., Tian, L., Jie, Z., Zou, Y., Lin, X., Liang, H., Li, W., Ju, Y., Qin, Y., Zou, L., Lu, H., Zhu, S., Jin, X., Xu, X., Yang, H., Wang, J., Zong, Y., … Zhang, T. (2021). Metagenome-genome-wide association studies reveal human genetic impact on the oral microbiome. Cell Discovery, 7(1). https://doi.org/10.1038/s41421-021-00356-0
- Park, S., Kim, C., & Wu, X. (2022). Development and Validation of an Insulin Resistance Predicting Model Using a Machine-Learning Approach in a Population-Based Cohort in Korea. Diagnostics, 12(1). https://doi.org/10.3390/diagnostics12010212
- Lundberg, S. (2019). SHAP (SHapley Additive exPlanations). Github Repository.
- Saeed, W., & Omlin, C. (2023). Explainable AI (XAI): A systematic meta-survey of current challenges and future opportunities. Knowledge-Based Systems, 263. https://doi.org/10.1016/j.knosys.2023.110273 Tiozon, R. J. N., Sreenivasulu, N., Alseekh, S., Sartagoda, K. J. D., Usadel, B., & Fernie, A. R. (2023). Metabolomics and machine learning technique revealed that germination enhances the multi-nutritional properties of pigmented rice. Communications Biology, 6(1). https://doi.org/10.1038/s42003-023-05379-9